What can psychology teach us about AI’s bias and misinformation problem?
When you're curious, you're most open to learning new things. But much-hyped AI systems providing wrong or biased answers could have serious repercussions for learning, psychologists warn
June 22, 2023
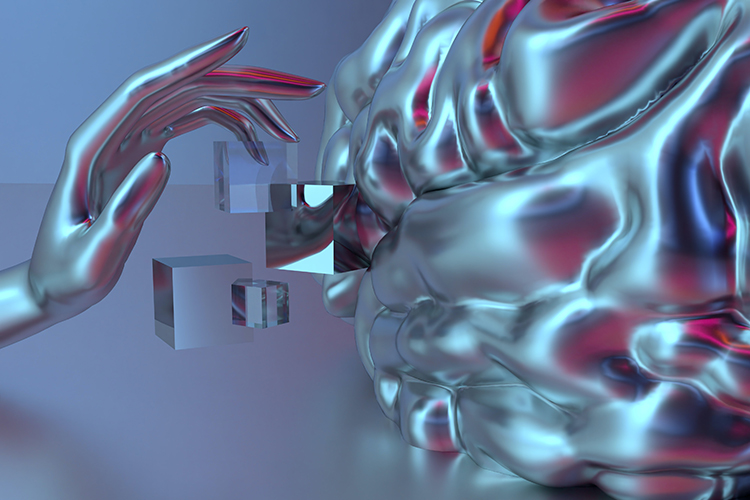
When humans are curious about something and seeking information, they’re more likely to remember what they learn. But as generative artificial intelligence tools like ChatGPT become more available and trusted, psychologists worry the wrong answers they provide will be treated as truth among users. That misinformation is incredibly difficult to correct. (Photo by Allison Saeng via Unsplash)
Knowledge may be power. But what if the information that leads to that knowledge is wrong?
To Celeste Kidd, assistant professor of psychology at UC Berkeley, the answer is simple: It’s dangerous and perhaps the most concerning aspect of generative AI’s rapid expansion.
Systems like ChatGPT, Stable Diffusion and DALL-E have rippled across the planet. Millions of people have used these platforms for amusement, incorporated them into their professional workflows and turned to them for quick answers on subjects both trivial and complex.
This AI boom has led thousands of scholars and technology leaders to call for a pause on future developments, citing what they call “profound risks to society and humanity.” From job disruption to a corporate AI arms race, the stakes are high.
But perhaps the most perilous and overlooked risk is how racial bias and outright falsehoods that AI systems churn out can forever alter what we know and how we think, Kidd said.
In a perspective published today in the journal Science, Kidd and co-author Abeba Birhane, a Trustworthy AI senior fellow at the Mozilla Foundation and adjunct assistant professor in computer science at Trinity College Dublin, explain how decades of psychological research into how we learn “can help build a bridge of understanding about what is at stake.”
“When company officials talk about the potential harms, they overwhelmingly give examples of nefarious forces using this technology for bad,” Kidd said. “In this piece, we’re talking about the harms that will happen from the intended use of these systems. It does not require a nefarious force to use the system in order to generate disinformation that’s intended to deceive people.”
Berkeley News spoke with Kidd about the commentary in Science, the state of AI, and what psychology can teach us about the risks posed by these platforms.
Berkeley News: You began drafting this piece in February, before many called for a pause on AI development. Why was it important for you as a psychologist to explain how AI distorts human beliefs?
Celeste Kidd: It’s been well established that these AI models produce biased material and also fabrications. That’s not new. What was disheartening for me and other people working in this area to hear was the developers’ responses: “Yes, there are problems,” they’d say. “There are distortions. There are fabrications and biases. But we need people to use the models in order to discover the problems, and then we’re going to correct them.”
The problem with that logic is that a lot of the biases and fabrications may not be detectable to people — especially if they’re using the system to help them make up their minds.
By that point, these systems have transmitted the information to the person. It may not be easy to correct.
In the piece, you mention several “tenets of psychology” that help explain why bias and misinformation are such important things to understand when it comes to learning. What do you mean?
We know that people form stronger beliefs more quickly on the basis of agents that they view to be confident. These chatbots are like a conversation that you’re having, which is very different from the outputs you might encounter when you’re searching online. This feels more like a person because of the nature of the presentation of the information.
Also, these systems don’t generate the kinds of uncertainty markers that a human agent would. When people are talking, they’ll say things like, “I think” or “I’m pretty sure.” There are all sorts of verbal markers of being unsure. Generative AI model outputs don’t have anything in them that signals uncertainty to let people know that the responses may not be trustworthy. In fact, there’s nothing in the architecture of these models that could be used to build in a signal of uncertainty in the outputs.
These models cannot discern fact from fabrication. That is a problem.
You also wrote that there’s a limited window of time when we’re open to changing our minds while learning new things, right? Tell me more about that.
If somebody is using something like ChatGPT in order to search for information, if they’re regarding it as a tool that indexes or catalogs all of human knowledge, they’re relying on it to provide them with good information. They’re coming into using that system at a very particular moment, which is when they’re very curious.
You have uncertainty, and once it’s resolved, the window to change your mind closes. It’s not easy to open after that fact.
– Celeste Kidd
When you’re very curious is when you’re most open to changing your mind. That’s when you’re looking for information. That’s when learning happens. It’s a magical time. But if that is the moment at which you are fed biases and fabrications, it’s a problem, especially if they’re conveyed confidently and especially if they are reinforced, if they’re occurring repeatedly. These systems are designed to present a sort of exhaustive, pithy response. That’s exactly the kind of information that we worry would close this opportunity of learning.
In other words, you have uncertainty, and once it’s resolved, the window to change your mind closes. It’s not easy to open after that fact.
You wrote that marginalized groups are among those most negatively affected by these biases. Recent reporting has also shown this in stark detail. How do you see this becoming a more entrenched problem?
People are constantly paying attention to statistics in the world and integrating those statistics into their view of how things in the world work. If you’re using Stable Diffusion, a text-to-image AI model, in order to generate images based on occupations, and there are stereotypes and biases in there, that is something that’s going to impact your own perception.
It’s important to be able to guard against these systems that wrongly suggest most criminals or drug dealers are people of color.
We’re living in a moment when things like misinformation and disinformation are already ubiquitous. People might be quick to lump generative AI in with the rest of the half-truths or lies that already swirl online. You’re saying it’s actually more concerning. Why?
The fact that you are interacting with these systems as though they’re agents is something different that we haven’t seen before. The fabrications are also very different from what we’ve seen with search. There’s nothing in these models that has the ability to discern fact from fiction.
The technology is amazing. It does some things really, really well. But there is nothing in that process that’s even looking for whether the material is true. This is very different from something like a search algorithm that’s indexing and recommending and ordering human-created content.
These systems will also fundamentally change the contents of the internet, so far less of it is human-created, creating cascading problems downstream that impact even traditional search algorithms. The model outputs — and all the fabrications and biases they contain — will subsequently be used to train future models, exacerbating these problems.

“How are people regarding these models?” Kidd asked. “How are they interacting with these models? These are things that can be studied and should be studied empirically so that we’re able to generate the most efficient course of action for how to mitigate the risks.” (Pexels photo by Cottonbro Studio)
In the past six months, it’s been hard to avoid news about these systems, including the doomsday scenarios. Is that part of the problem you’re outlining, too?
There’s a lot of hype around these systems. There’s a lot of media coverage that is being pushed by the companies and people that have financial interests in creating the perception that these are very sophisticated technologies.
That hype in and of itself could actually do more harm than the systems would by themselves.
Because of that hype, people come into these systems expecting them to have human levels of intelligence. They may be more rapidly swayed in ways that are more permanent than if they were aware of the truth, which is that these systems are not that smart.
You write about how those misinformed beliefs are then passed from one person to another in perpetuity. That’s not very hopeful.
It is a dismal tone. I think that is the risk if these systems are widely integrated into lots of other things. That creates the opportunity for repeated exposure to the same kinds of fabrications and biases. If many people in a population are using the same system again, that’s a problem. Because one of the real strengths of humans as a species is our ability to rely on one another and the variance in terms of people’s opinions.
It does not require a nefarious force to use the system in order to generate disinformation that’s intended to deceive people.
– Celeste Kidd
Sometimes a lot of things in the world are really hard to know. What is the meaning of life? What should I be doing with my time on Earth? Those are big questions that are unknowable. And as humans, for those kinds of questions, we survey people. We’re paying attention to what other people think. We are updating as we encounter a wide variety of different kinds of opinions.
The fact that when you’re collecting information in that way, in a context where there’s noise, where there’s a lot of differences of opinions, it does make it harder. It’s less satisfying because you don’t get that little nugget of just the information you’re seeking. You don’t just get a simple answer.
But that’s good in the context of things that are difficult to know or things that are changing. If you don’t get that satisfying little nugget, you remain curious. And that means that you remain open-minded to integrating new information as it unfolds over time.
You offer some suggestions on a path forward. What are those?
One of them is resources to develop materials to inform policymakers and the public about what these systems are and are not. That has to happen, and that mission has to be led by people that don’t have a financial interest in these models doing well.
I would call for a pause to the hype. That’s the most important and most urgent thing.
– Celeste Kidd
We also need resources urgently for studying how things like your perception of confidence in a model impacts the degree to which it’s able to distort your beliefs and the degree to which bias is transmitted to you. We know what to expect, generally, from decades of psychology research.
That aspect of it, the fact that people are influenced by the material that they encounter, is not new, but there are a lot of variables that we’re missing. So how are people regarding these models? How are they interacting with these models? These are things that can be studied and should be studied empirically so that we’re able to generate the most efficient course of action for how to mitigate the risks.
You were thinking about this piece months ago, before some people came out and called for sort of a pause on the development of these tools. Do you consider yourself somebody who also thinks that there needs to be a pause?
That’s a tricky question. I don’t think I want to answer it in exactly that format. I will say something, though. Some of the themes of the Science piece appeared in both my and Abeba Birhane’s earlier work. We have a longstanding history of showing concern for biases in systems and the ways in which they might spread among and impact people.
The hype around generative AI at the moment makes those issues much more urgent. And it makes the distortions potentially worse, because, again, it leads people to believe that this is something that’s really smart. This might lead you to believe this is something that can be trusted.
I would call for a pause to the hype. That’s the most important and most urgent thing.