Turning cars into robot traffic managers
Self-driving cars may one day do more than just get us from point A to B
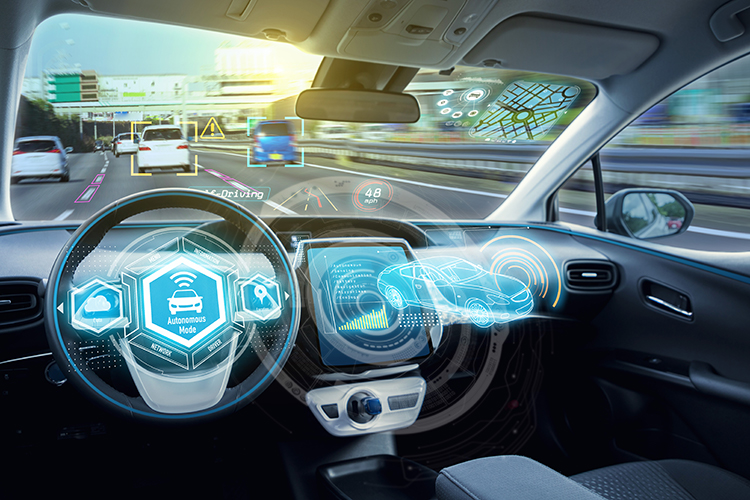
November 2, 2018
Self-driving cars may one day do more than just get us from point A to point B. By adjusting their speed and position while they merge, or by pacing their average speed on the road, they could also help reduce the bottlenecks and random slowdowns on busy thoroughfares like the Bay Bridge, getting us where we’re going faster and more efficiently than if we all drove ourselves.
UC Berkeley transportation engineers are developing a tool that uses a type of artificial intelligence called deep reinforcement learning to help autonomous, semi-autonomous and human-driven vehicles share the road. The project, called Flow, presented its first proposed standards for solving real-world traffic problems this week at the Conference on Robotic Learning in Zurich, Switzerland.
“Flow solves large-scale, multi-vehicle problems by using simulations that are much more efficient than what can be produced without the aid of artificial intelligence,” said electrical engineering and computer sciences professor Alexandre Bayen, director of the UC Berkeley Institute of Transportation Studies and the study’s principal investigator. “And we’ve made it a cloud-based, open-source system so the development community can continue to build on it.”
The proposed standards give researchers benchmarks for comparing different traffic management algorithms. For example, in one benchmark or “task,” featuring a computer model of the Bay Bridge, researchers can test to see how quickly their algorithm gets cars off the bridge — and more importantly, how it stacks up against similar algorithms.
“Unless we’re working on the same problem, it’s hard to compare results. Are you looking at a New York highway or a California freeway? A group of 20 cars or 50? You need an apples-to-apples comparison to understand which solution works better,” said Eugene Vinitsky, co-lead author on the study and Ph.D. student in Bayen’s lab.