UC Berkeley historian of science ponders AI’s past, present and future
"AI will make our lives better. But AI will also have downstream consequences that we have just the earliest inklings of," Cathryn Carson, chair of UC Berkeley's history department, said in a Q&A.
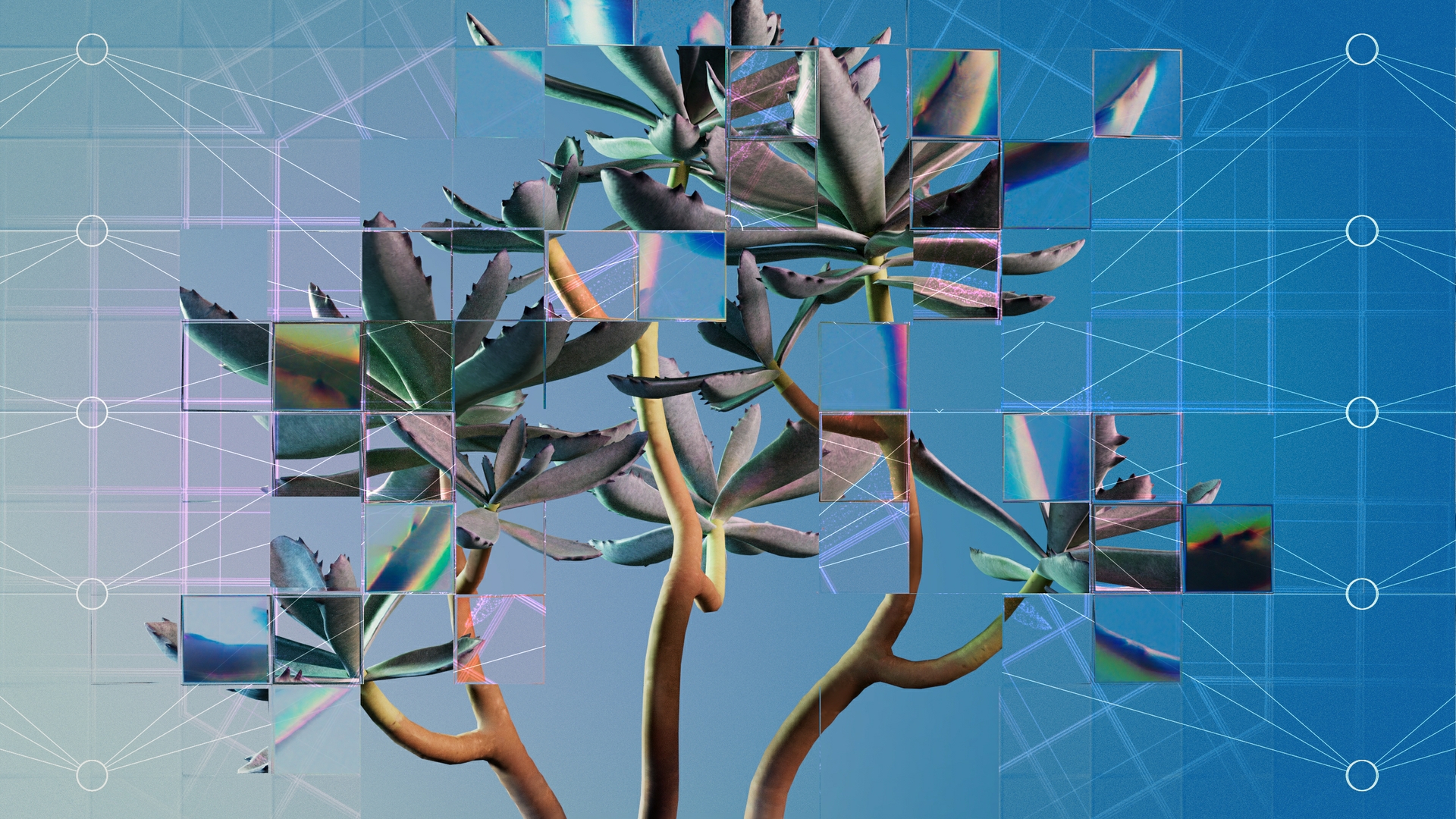
Alan Warburton via Wikimedia Commons
September 11, 2023
It doesn’t take long for a conversation about artificial intelligence to take a dark turn.
New technology presents a greater threat than climate change, some have said. It poses a risk of extinction. And the analogy du jour: AI development is akin to the race for nuclear weapons, so regulation should follow templates in line with the Manhattan Project or the International Atomic Energy Agency. The latter juxtaposition is so common that The New York Times recently published a quiz with the headline: “A.I. or Nuclear Weapons: Can You Tell These Quotes Apart?”
Convenient as the comparisons may be, it’s more complicated, said Cathryn Carson, a UC Berkeley historian of science who has studied the development of nuclear energy and the regulatory policies that followed. The risks are new, Carson said. But the way people and regulators respond is following a familiar set of moves thats unfolded repeatedly in recent years.
As tech companies have gained more power and prominence, historians like Carson have argued for their work to serve as a guidebook.
That way, we don’t make the same mistakes.
“The only way we can understand how the present works is by looking at the actors and the forces at work and how they relate to each other,” Carson said. “And then combining that with understanding where the present came from and what momentum it carries.
“How you could do that without history, I wouldn’t know.”
Berkeley News spoke with Carson about the current moment in our technological trajectory, what history might teach us about demands for change and government oversight, and why a better analogy might be to plastics and fossil fuels rather than the race for an atom bomb.
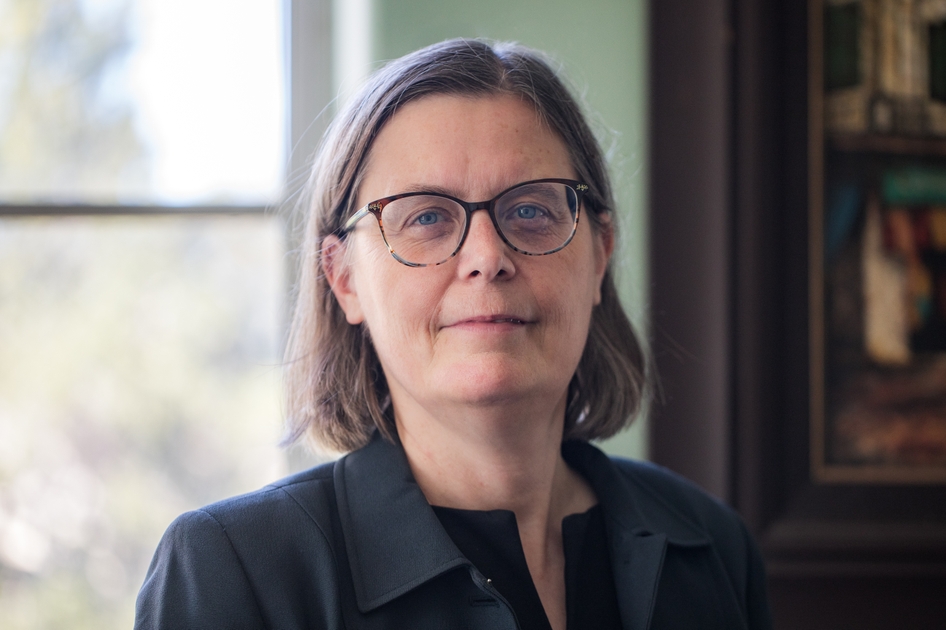
Brandon Sánchez Mejia/UC Berkeley
Berkeley News: Assuming there is some merit to the adage that history doesn’t repeat, but it rhymes, what is surprising to you about the current state of AI and machine learning?
Cathryn Carson: The technical developments in this field are fascinating, and they’re coming fast and furious. And sure, there are surprises around the corner. Generative AI is going to continue to astonish us. Automated data-driven decision-making is going to reach into new areas of life and the impacts are yet to be foreseen.
Societal dynamics around AI and machine learning surprise me less. They’re grounded in really familiar processes. Technical development, marketing hype cycles, free trials followed by paid premium services, and products released into the wild without all of the edge cases worked out. Data expropriation. Demands for regulation. Stories of individuals harmed by these products.
Those aren’t hard to predict until our society changes.
What do you mean by that?
If we want a world in which AI works to serve people more broadly — besides those actors who have the resources and the power to create it — that means figuring out how things can shift away the concentrations of power and resources in the hands of people who already have it, or who can purchase AI-based services and use them for their own interests.
If we want a world in which AI doesn’t reproduce the patterns and structures of advantage and disadvantage that are already rife in our society around wealth, status and empowerment, or around oppression and exploitation, then we need to actually set up mechanisms that actively work against reproducing them.
People say we need to correct biased training data, and we need to put diverse teams to work on AI. Yes, we need to do that. But it goes beyond that.
We have to acknowledge that AI and machine learning and automated decision systems are built on data that are extracted from people and communities, usually without their full knowledge, sometimes — maybe often — against their interests. Those systems are then used to govern those folks and shape their decisions and outcomes and opportunities and serve other people who make the systems and run them.
So if we want things to be different in the future from what we’re seeing now, that’s the dynamic to go after. To get the people who are affected by the technologies into a position to speak back powerfully and effectively to those who made these systems and shape what they’re enabled and permitted and built to do.
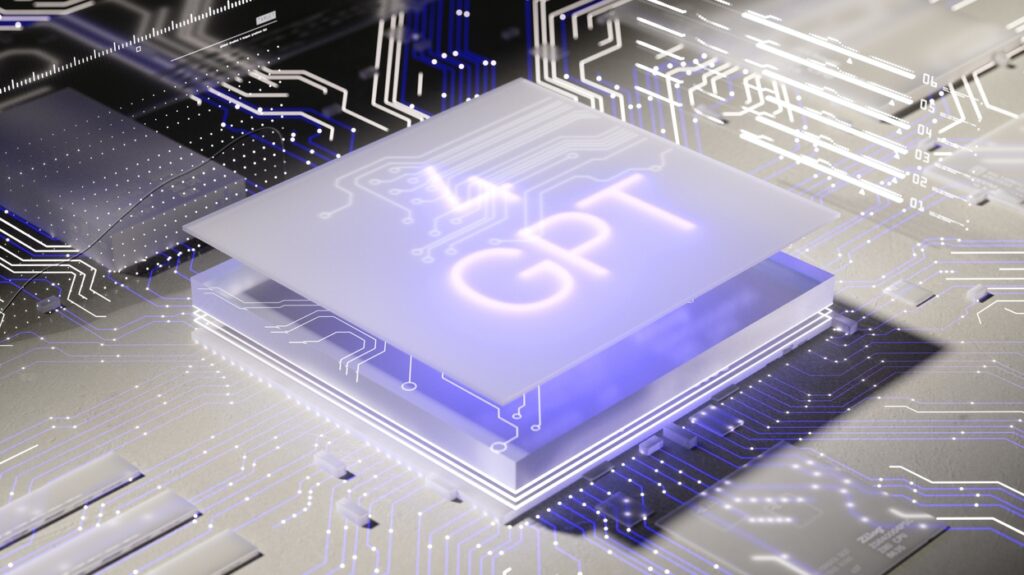
Is it surprising to you how seemingly blasé many people have become with these concerns you’ve expressed about data, privacy and private, for-profit companies?
These are hard changes to make or even imagine making. There’s a tendency to say, “It couldn’t be otherwise than what we have now, so I might as well just accommodate myself to it.” I encounter this with students a lot. Helping them think their way through it is integral to how we do data science at Berkeley. At the undergraduate level, we take them through these questions: Why does our world work this way? Why is our data harvested and used to build products that are then pitched to us, used to track us and surveil us? When did we ever agree to this? What on Earth can I do about it?
The only way you come to see that there might be an alternative is by understanding how we got to where we are now. Understanding, for instance, that we work under a data protection regime that was essentially designed in the 1970s, when corporations were not a major concern, and the speed of datafication was faster than it had been before, but not nearly as fast as now.
The scale of the problem is such that it has to be approached systemically rather than simply in making pretty small and ineffective personal choices.
Cathryn Carson
Once you realize we’re operating under a regulatory regime that has been amended around the edges but is still essentially a 50-year-old project, you start seeing how the place to work here is to undo some of the larger societal assumptions — assumptions like how innovation must be advanced or how downstream costs are relatively less important.
You have to go to the root cause of the problem rather than being focused solely on questions like creating better terms of service or clicking the right links on the website so that my data isn’t collected. You realize that the scale of the problem is such that it has to be approached systemically rather than simply in making pretty small and ineffective personal choices.
From a policy perspective, what needs to be front and center of conversations and ultimately meaningful changes to overcome these things that you’re highlighting?
Systemic change is hard, not just because it’s complex, but because it’s resisted. I would think about the challenges that are in front of us, when it comes to changing how our society allows data to circulate, as being in a way comparable to other challenges, like challenges around fossil fuel use and climate change. Or challenges around our dependence on plastics and the impacts downstream that we’re now seeing. Just because problems are hard doesn’t mean we don’t get started.
But it does not mean addressing challenges of climate change and fossil fuel use through just individual adjustments to our thermostats or by only shifting individually from plastic bottles to refillable containers. Those are very small parts of the solution. The larger parts of the solution come with the hard negotiations about what is permitted to happen, who benefits from it, and who pays for it. In that sense, the regulatory arena is a key place to operate. That’s the place that actually has the capacity to change the power relations that are underlyingly responsible.
You’re an expert on 20th century policy on nuclear power and weapons. What parallels do you see between then and now?
I was trained as an historian of physics and the nuclear era. I’ve done research, and I’ve taught about physicists’ encounters with state power in relation to nuclear weapons and also nuclear technologies. And now, Oppenheimer is back on our minds.
We are… so focused on what we’re seeing right now and the near term that we’re not yet equipping ourselves to think deeply about the structural challenges in the long term.
Cathryn Carson
There are a lot of proposals out there to manage AI like it’s assumed nuclear fission was — either a Manhattan Project for AI safety, gathering huge cadres of technical experts to solve the problem, or an international regulatory agency that could set global guardrails on development, like the International Atomic Energy Agencys focus on safeguards and peaceful uses.
I see why both of those references from the 20th century nuclear era are analogies ready to hand. The people who launch them are grasping for a response that goes some way toward capturing the magnitude and acknowledging the stakes of what they see coming.
But they’re also reaching for responses that are familiar and that steer things in directions that they’re probably more comfortable with — regulatory bodies that still allow a lot of space for commercial development, while taking the edge off of the fear.
As a nuclear historian, what stands out for me as similar to the current age of AI, rather than those particular limited analogies to the nuclear era, are the political stakes and the contestation that comes with high-stakes political power.
You mean the difference between governments leading the development charge then versus for-profit companies now?
Yes. Because the political actors around machine learning and AI today are not primarily — they’re not even simply — the nation-states that had a monopoly on nuclear fission. That was a crucial aspect of nuclear power, that only nation-states, and only a handful of them, had the capacity to build nuclear weapons.
Today, the political actors are just as much the corporations building and extracting value through AI technologies, and through data technologies more generally. That’s a pretty crucial difference from the nuclear era: the central role of corporations, tech companies and other companies that generate and extract value, capture markets, draw resources and power to themselves through AI.
Are the stakes higher now than they were then? Or are they just different stakes?
I think they’re more comparable, honestly, to the stakes of plastics and fossil fuel refining. I tend to find more power thinking with those analogies than with some forms of existential risk thinking that comes out of comparing AI to nuclear weapons. Instead, it’s sort of the mundaneness, the routineness of technological transformations linked to plastics or fossil fuels. And the fact that they’re embedded within corporations and markets as much as or more than nation-state actors.
Yes, AI will make our lives better. Plastics did, too. They will make some corporations quite powerful and rich. But AI will also have downstream consequences that we have just the earliest inklings of.
And we are, for the most part, so focused on what we’re seeing right now and the near term that we’re not yet equipping ourselves to think deeply about the structural challenges in the long term.
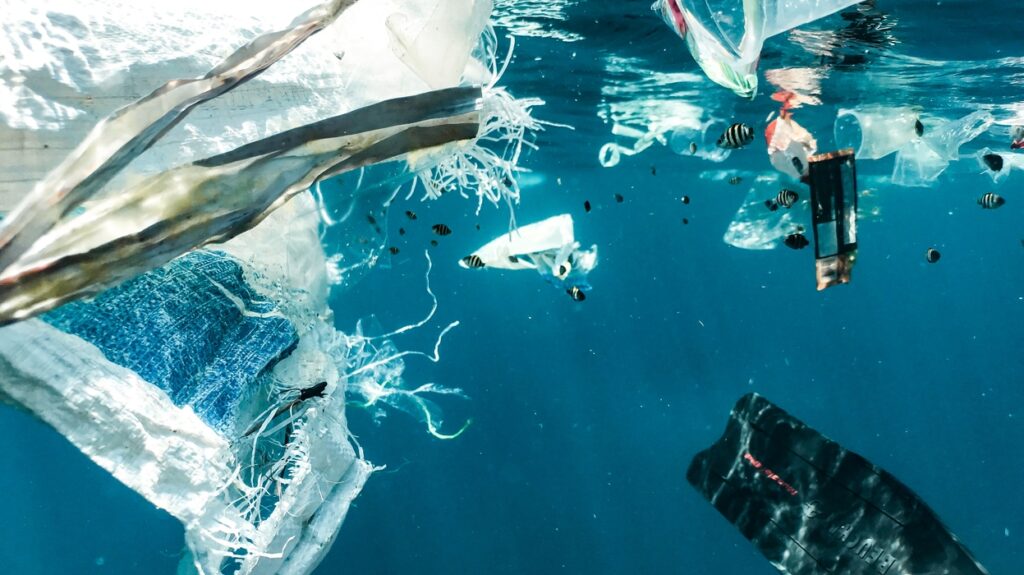
There seem to be two distinct camps of people most involved in conversations around AI right now. There are those in the industry and end users who love it. And there are doomsayers who argue that it poses a particularly grave threat. It seems like youre arguing for some sort of middle ground?
There’s a third position between the unsurprising advocacy of those building and benefiting from these models and the sort of long-term fear that this brings us to doomsday. That third position is mostly occupied by folks who move back and forth between the social sciences and technical expertise, trained in different disciplines like science, technology and society or history, law, sociology or critical theory. Folks who work in the present day with an eye to both the past and the future in really concrete, realistic terms and who are not themselves trying to make a case for the technologies or dismiss them as frightening and out of hand. Folks who want to understand — and act on that understanding — how we can use social scientific concepts and tools in order to work on the present, to address the fact that these technologies are here, are being developed, are impacting people now already.
That time horizon of working in the now with the people who are here now is really different from thinking about what might happen years down the line when machine learning tools and AI are more equipped than they are. You work in the present because that’s where you can set good social relations. That’s where you have the capacity to shape things in the now.
This third group of people tends to be demographically different from either of the first two groups. The presence of people of color and women in that third group compared to their relative absence in the first and second is pretty striking. When you watch what plays out in The New York Times, you see the first and second groups.
Where much of the intense work is being done now is in the third group.
Are you hopeful that we, collectively, are capable of finding a path for the answers you’ve outlined?
I approach hope through my teaching. I’m fortunate enough at Berkeley, together with my colleagues in data science, to teach literally thousands of students a year. And that platform, that scale that Berkeley provides, is where I get my hope.
Questions that are this big are not going to be solved by individual geniuses. They’re going to be solved by a collective effort of people with a diverse set of skills and who know enough about each other’s areas to respect what others bring. I find it really exciting and just hugely inspiring, in a way that sort of chokes me up, that Berkeley has invested in a data science program like this, and that I have colleagues here in computer science and statistics who partner with me to allow us to train brilliant Berkeley undergraduates and give them tools for historical thinking, social scientific analysis, as well as the computational and inferential skills that we teach them.
To me, that’s where the hope comes from. It’s not from anything I will do, and it’s not from any technical solution that will come out. It’s that Berkeley has made itself a platform for our students to be part of the solution.